Understanding the Importance of Labeling Tools for Machine Learning
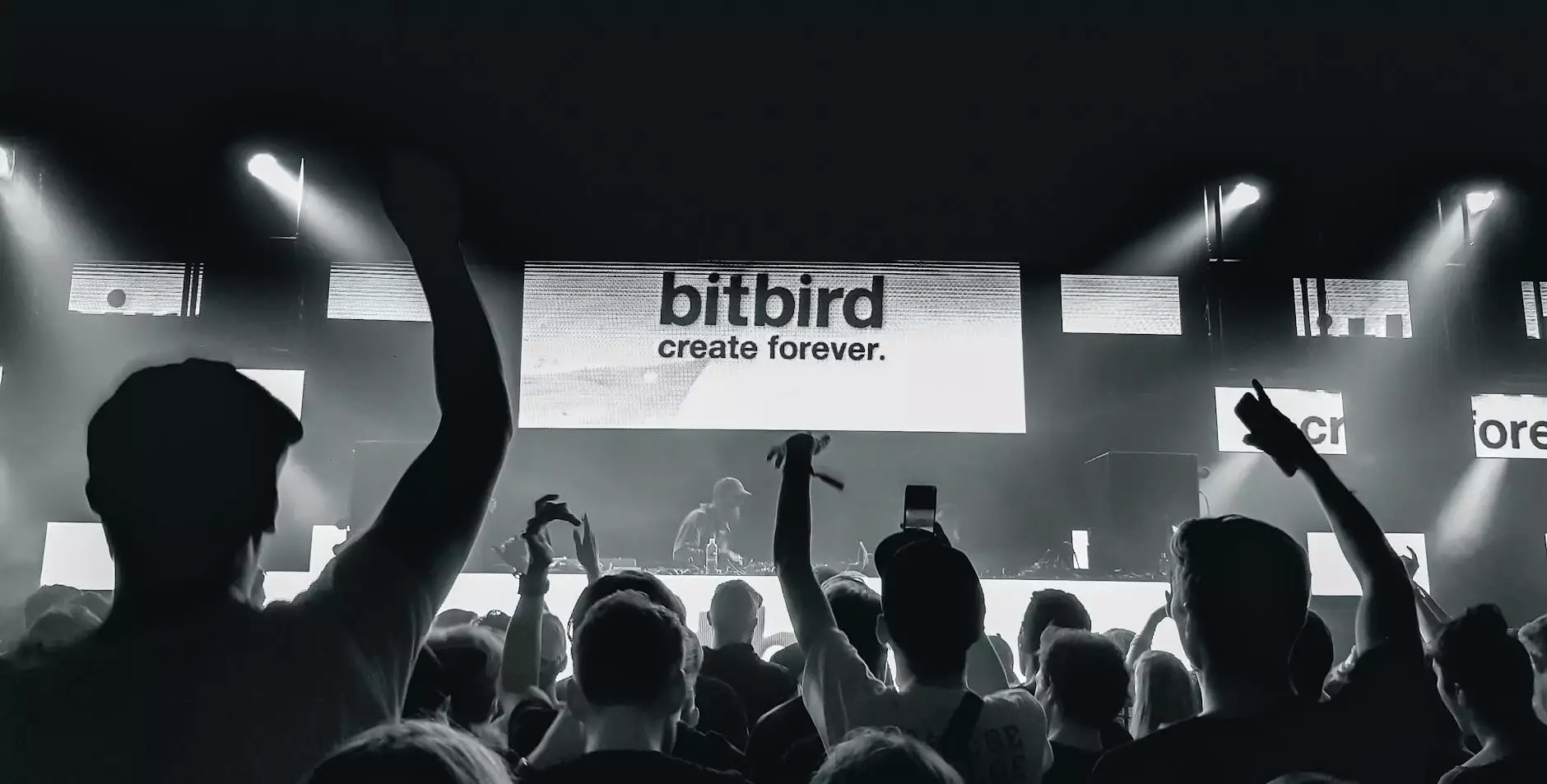
In the rapidly evolving landscape of artificial intelligence and machine learning, labeling tools for machine learning have become essential components for organizations looking to harness the power of their data. These tools are pivotal in creating and preparing datasets that enable machines to learn from human input, which in turn leads to improved decision-making and enhanced operational efficiency. In this article, we will explore the various depths of labeling tools, their significance, and their impact on business, specifically in the realms of Home Services and Keys & Locksmiths.
What Are Labeling Tools for Machine Learning?
Labeling tools for machine learning are software applications designed to help users annotate and label data which can include text, images, videos, and audio. Labeling is a critical step in the data preparation phase for machine learning tasks, where the objective is to teach models how to recognize patterns and make predictions based on data provided.
The Types of Data Used in Machine Learning
Below are some of the key types of data used in machine learning which require effective labeling:
- Image Data: Used in applications like facial recognition, image classification, and more.
- Text Data: Essential for NLP (Natural Language Processing) tasks like sentiment analysis and document classification.
- Audio Data: Important for voice recognition and sentiment analysis from speech patterns.
- Video Data: Used in surveillance, behavior detection, and various interactive AI applications.
Why Are Labeling Tools Crucial for Businesses?
Businesses that operate in sectors such as Home Services and Keys & Locksmiths can derive significant benefits from using sophisticated labeling tools for machine learning. These advantages include:
Enhanced Accuracy in Machine Learning Models
The quality of the labeled data directly influences the performance of machine learning models. Accurate labels lead to higher precision and recall values in model predictions, ultimately resulting in better outcomes for businesses. With the right labeling tools, companies can ensure that their datasets are correctly annotated, which improves model reliability.
Streamlined Data Preparation Processes
Labeling can often be a time-consuming task. However, advanced tools can automate and simplify the process, making it much more efficient. Features such as bulk labeling, collaboration tools, and intuitive interfaces enable teams to annotate data swiftly, allowing businesses to move faster in deploying machine learning solutions.
Cost-Effectiveness
Investing in quality labeling tools may seem expensive at first glance, but the long-term savings derived from increased operational efficiency and reduced error rates can result in significant cost savings for a business. For Home Services and Keys & Locksmiths, this means that resources can be allocated effectively, enhancing profitability.
Choosing the Right Labeling Tools for Machine Learning
When selecting labeling tools for machine learning, businesses must consider several factors to align the tools with their specific needs:
1. User-Friendliness
The accessibility of the tool for users of various technical backgrounds is essential. A user-friendly interface reduces the training time and allows teams to focus on labeling rather than navigating complex software.
2. Integration Capabilities
Choose labeling tools that can easily integrate with existing machine learning frameworks and data management systems. This includes compatibility with platforms like TensorFlow and PyTorch, as well as data storage solutions.
3. Scalability
As your data volume grows, your labeling tools should be able to scale accordingly. Look for tools that can handle large datasets without a drop in performance.
4. Support for Various Data Types
For businesses engaging in diverse projects, it is vital to look for tools that support multiple data formats, including text, images, audio, and video.
5. Cost and Licensing Options
Analyze pricing structures and licensing options to ensure the tool fits within your budget. Explore free trials and tiered pricing that allows for flexibility as your business grows.
Top Labeling Tools for Machine Learning
Now that we understand what to look for in labeling tools, let's explore some of the leading solutions available in the market:
A. Labelbox
Labelbox is a powerful data labeling platform that streamlines the process through automation and allows for easy collaboration among teams. The tool supports various data types and provides a user-friendly experience, making it a favorite among businesses.
B. Prodigy
Prodigy is an annotation tool that focuses on efficiency and improves decision-making by allowing users to incorporate feedback into the labeling process quickly. It is well-known for its adaptability and integration with machine learning workflows.
C. Supervisely
Supervisely is ideal for working with images and video data. Offering robust tools for annotation and management, it is particularly useful for projects that involve computer vision. This is especially valuable for businesses that require precision in visual data interpretation.
D. Snorkel
Snorkel takes a different approach—using weak supervision to create labeled datasets without extensive manual effort. This tool is suitable for businesses like Keys & Locksmiths that may have limited resources but still require high-quality labeled data.
Implementing Labeling Tools in Your Business Strategy
Integrating labeling tools into your business strategy begins with identifying specific use cases. Here's how you can evaluate and implement these tools effectively:
1. Define Your Objectives
Understand why you need machine learning and how labeling tools will facilitate achieving your goals. This will help you tailor your approach to meet business needs.
2. Train Your Team
Invest time in training your team to ensure they are well-versed in using the labeling tools you select. Provide comprehensive resources and encourage an environment of continuous learning.
3. Monitor Performance and Iterate
Once your labeling processes are in place, continuously monitor the performance of your machine learning models and iterate on your labeling strategies. Feedback loops will enable you to enhance data quality and overall business intelligence.
Conclusion
In conclusion, labeling tools for machine learning are not just useful but essential for businesses, particularly in data-driven industries such as Home Services and Keys & Locksmiths. By investing in the right tools, companies can enhance their operational efficiency, accuracy in data predictions, and overall profitability. The future of machine learning is bright, and with the right labeling strategies, businesses can position themselves at the forefront of innovation and success.
As the demand for powerful data-driven insights continues to rise, the adoption and mastery of labeling tools will undoubtedly offer businesses a competitive edge. Start exploring your options today to harness the true potential of your data!